Algorithm Charter for Aotearoa: Six things to be doing now
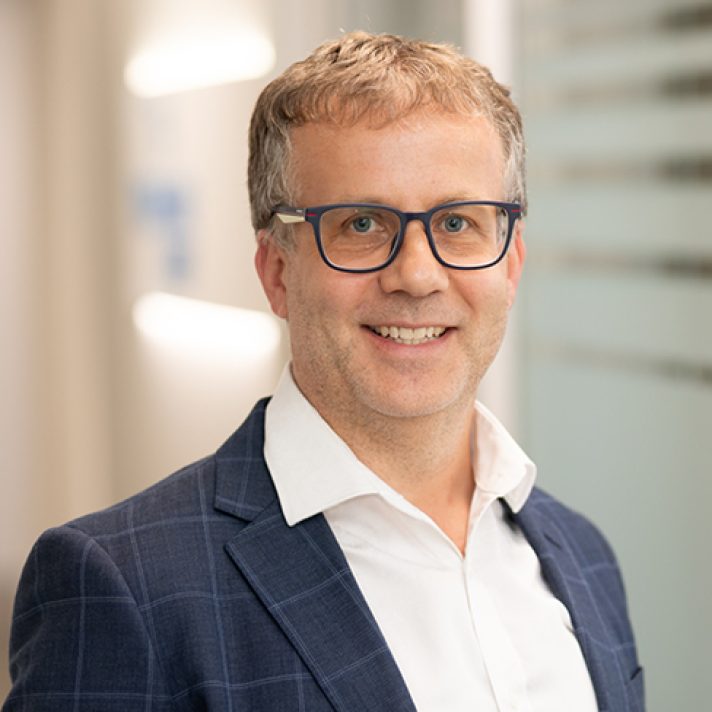
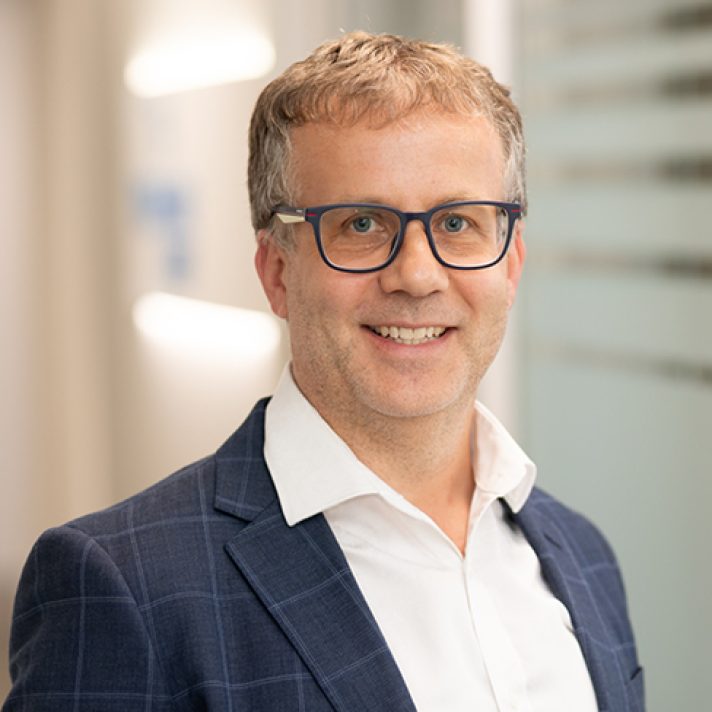
The New Zealand Government has recently released its Algorithm Charter for Aotearoa New Zealand. Effectively, the Algorithm Charter is a call to action for government agencies to provide New Zealanders with the confidence that algorithms are being used sensibly and ethically. We look at how government agencies can use the Charter to drive the effective and efficient delivery of government services in New Zealand.
Developments in computing have enabled ever increasingly sophisticated algorithms to support decision-making in all facets of our life. Algorithms are sequences of steps used to solve problems and when paired with large underlying databases for training, algorithms can help us plan and guide our lives. Alongside new playlist recommendations and advertisements for things we never knew we needed, algorithms are increasingly responsible for improvements in the quality of services offered by governments to their citizens. There are a wide range of operational functions already being guided by algorithms in New Zealand, including elective surgery prioritisation, allocation of Work and Income clients to services, and youth offending risk screening. Stats NZ’s 2018 Algorithm Assessment Report provides a useful stocktake.
As New Zealand government organisations scale up their use of algorithms, public servants are rightly nervous about getting it right. With great power (and highly sensitive administrative data) comes great responsibility, and highly publicised overseas government algorithm fails (for example, policing algorithms were judged to be racist) are front of mind for many.
What are the major risks when using algorithms for government decision making?
Algorithms encompass simple techniques, such as regression models and decision trees (which can be used to make predictions and streamline business processes), through to more complex approaches like neutral networks and ensemble models (closer to machine learning). Ideally, they streamline processes, make predictions, and reveal insights about problems in a way not possible for the human mind alone. However, if algorithms are not built with care they can be ineffective, or at worst dangerous.
The major risks to be aware of when using algorithms for government decision making need to be clear. These include:
Unfairness
The data used, or the way the algorithm is built can result in some sectors of society being unfairly targeted (or not targeted) by the services informed by the algorithm. For example, earlier this year, an African American man in Michigan was wrongfully arrested and held in a detention centre for nearly 30 hours after facial recognition technology incorrectly identified him as a suspect in a shoplifting case. Relatedly, the U.S. Department of Commerce’s National Institute of Standards and Technology’s Face Recognition Vendor Test looked at 189 software algorithms from 99 developers and found higher rates of false positives for Asian and African American faces relative to images of Caucasians.
Government agencies need to be particularly sensitive to ensuring the fairness of their algorithms, as they must meet human rights legislation which prohibits discrimination on the grounds of gender, sexual orientation, religious and ethical beliefs, colour, race, ethnicity, disability, age, marital status, political opinion, employment status and family status. However, satisfying ‘fairness’ criteria across all of these measures at the same time may prove challenging, and organisations will need to understand the trade-offs and make clear determinations on which they are adhering to, and why.
Ensuring fairness may mean some variables need to be restricted from being used as inputs to an algorithm, but grey areas remain. For example, if ‘gender’ is restricted, a secondary variable used as an input, such as ‘hobby’, might be strongly correlated. So as a result, the algorithm could inadvertently discriminate based on gender anyway.
Accountability
How algorithms inform decisions may not be clear, meaning that biases may go undetected. This makes clear lines of accountability critical. Without clear accountability, the safe implementation of an algorithm will be jeopardised. Machine learning and AI algorithmic techniques are particularly prone to ‘black box’ executions, where deciphering how the algorithm works is difficult. But it doesn’t have to be this way. Interpretable ‘glass box’ algorithms that facilitate strong lines of accountability are entirely feasible when the right techniques are employed. Our recent article, Interpretable machine learning: what to consider discusses these issues in more detail.
Lack of transparency and consequently accountability were an issue for Microsoft when, in 2016, it launched a chat bot on Twitter called Tay. It was programmed to learn how to interact like a real human, through reading and processing real tweets. However, human oversight was lacking as the black box model evolved and within hours it transformed from an innocent bot into an offensive, loud-mouthed racist.
Ongoing alignment with rules and policy
Periodic review of algorithms to ensure they remain fit for purpose may not occur, and human oversight, an essential component in protecting against sub-optimal outcomes and perverse incentives, may not be retained beyond an initial implementation period. While algorithms can inform targeting of services and save time, they need to align with the problem to be solved and should be updated as the broader environment evolves. The Australian’s Government’s ‘Robodebt’ saga is a cautionary tale. The Online Compliance Intervention was an automated debt recovery program introduced in mid-2016 in an attempt to ensure recipients of welfare benefits were not under-reporting their income and, as a result, over-receiving welfare payments. Almost half a million Australians received correspondence regarding overpayments, but hundreds of thousands of the assessments appeared wrong, because the algorithms had included income from other periods, where people were actually in paid employment and not claiming benefits. The Australian Government is now paying refunds to all 470,000 Australians who had their debt calculated using this income averaging methodology. Human oversight is critical to the safe operation of algorithms and a social licence to do so.
The issues we’ve briefly highlighted are all inter-related. Algorithms need to get it right, every time, because any single issue can erode public confidence irrevocably, no matter how effective the algorithm is.
Where does the Algorithm Charter for Aotearoa fit in?
Recognising these risks of mismanagement, the New Zealand government recently released the Algorithm Charter for Aotearoa New Zealand. It positions New Zealand as a world leader in setting standards to guide the use of algorithms by public agencies. Many government agencies have already signed up to the charter. The charter includes several commitments designed to ensure the appropriate management of the risks outlined above.
What should agencies do to ensure they are implementing the Charter well?
The Algorithm Charter for Aotearoa New Zealand is an enabler. By abiding by standards of fairness and transparency set out in the charter, government agencies can improve public confidence in the use of algorithms in government decision making. Without it, the use of algorithms may continue to be met with community resistance. Ideally, greater public trust, built on the foundations of this charter, will lead to greater use of algorithms (and leveraging of data more broadly), and ultimately more effective and efficient government services. A win for service users, communities and government agencies alike.
We outline six things government agencies can do to position themselves well for implementing the charter:
- Review your services and identify which are informed by algorithms.
Government agencies should perform a stocktake of all algorithms that are informing operational decisions. In most cases the relationship between a service and an algorithm is direct, for example, an algorithmic tool that informs the prioritisation of patients for surgery. However, sometimes the relationship may not be direct, such as when output from one algorithm is hard coded into another. Expert advice may be beneficial to support a stocktake.
- Prioritise algorithms for review.
The Algorithm Charter offers an easy rule-of-thumb approach, suggesting government agencies rate each algorithm based on:
- The likelihood of unintended adverse outcomes arising from use of the algorithm
- The impact that unintended adverse outcomes would have on New Zealanders
This rule of thumb is useful for an initial high-level prioritisation, however judging the likelihood of unintended adverse outcomes may be more difficult, particularly where the algorithm lacks transparency. In such cases, it will be beneficial to gather together subject matter and technical experts to work through the implications.
- Peer review (in priority order) algorithms against the Algorithm Charter commitments.
Peer reviews should be thorough. Identifying bias in algorithms can be the most difficult, but arguably the most important, aspect of a review.
Public confidence in any review is likely to be enhanced if it is independent from the creators and end-users of the algorithm.
- Adjust algorithms where they do not meet Algorithm Charter commitments fully.
Modern algorithmic techniques can be employed to address most issues. Transparent, interpretable algorithms are entirely possible. And while building public confidence in the use of algorithms may take time, it is an attainable outcome if charter commitments are adhered to.
It will be beneficial at this stage to think about what might be considered fair or unfair by society in terms of the way the algorithm affects decisions. Any adjustments to algorithms will need to be designed with fairness in mind.
Releasing public advice that a review has been undertaken and that some algorithms have been adjusted as a result will help make it clear that algorithms are being effectively monitored under the charter.
- Put in place a periodic review process for each algorithm.
The regularity and depth of periodic reviews for each algorithm should be commensurate with the assessed likelihood and impact of unintended adverse outcomes. Your organisation should also have a management plan in place for its algorithms in the event they result in unintended consequences for New Zealanders. The plan should become operational as soon as issues are identified.
- Set up guidelines for the development and management of new algorithms.
The Algorithm Charter puts in place generic commitments. Each government agency will need its own set of more detailed guidelines for new algorithms. These will need to reflect the nature of the services a particular government agency provides, the sensitivity of service users to the use of algorithms, and the use of their data to inform them. For example, high sensitivity over the use of personal health data may dictate specific guidelines relating to the use of algorithms in the health sector.
Embrace the opportunities presented by the Algorithm Charter
While the scale of implementing the Algorithm Charter for Aotearoa shouldn’t be underestimated, there is a substantial upside to getting it right. Increased public trust in governments using algorithms for good should provide decision makers with more confidence to identify new opportunities to improve services using algorithms. This would be of particular benefit in fields where lack of confidence has previously prevented their use.
Other articles by
Daniel Stoner
Other articles by Daniel Stoner
More articles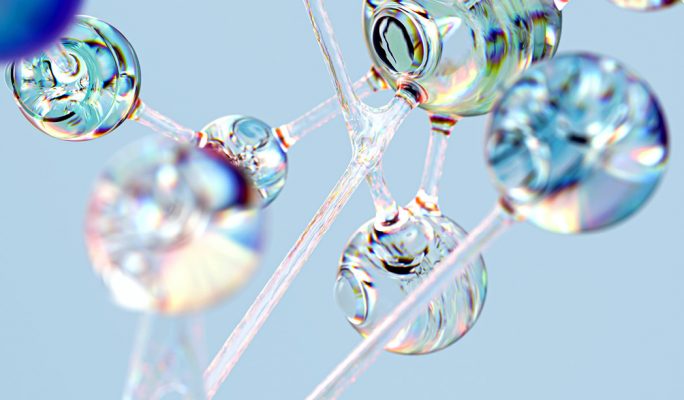
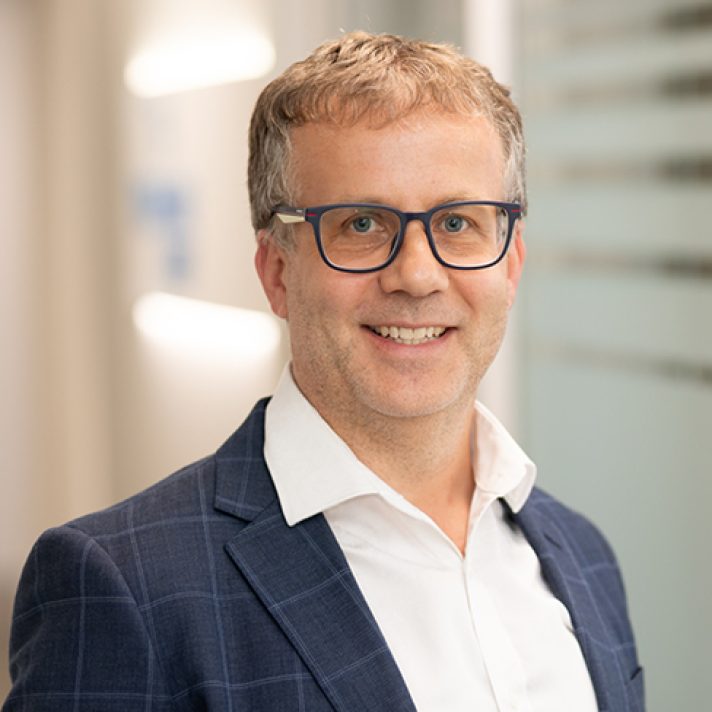
Lessons learned one year on – Algorithm charter for Aotearoa New Zealand
Take a look at the lessons learned from Taylor Fry's newly released review of the Algorithm charter for Aotearoa New Zealand
Read Article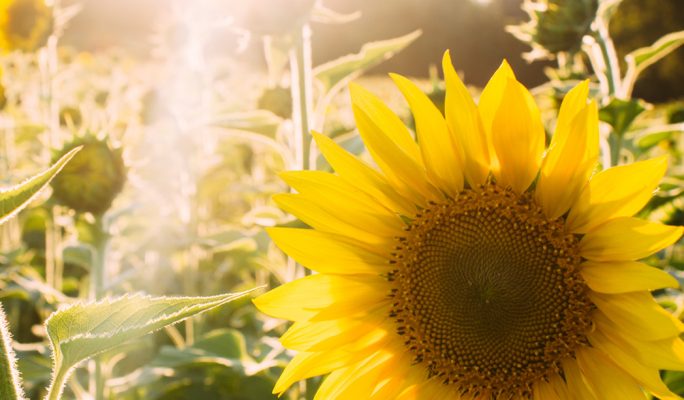
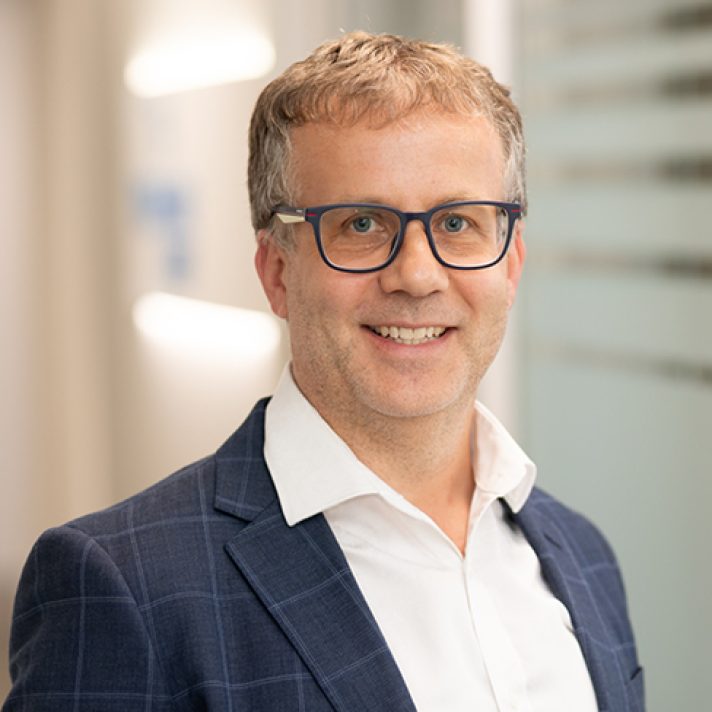
Analytics quick wins: Five New Year resolutions to thrive in a climate of economic uncertainty
How can advanced analytics help you through the COVID-19 storm? We reveal some ways to strengthen your business and prepare for success
Read Article
Related articles
Related articles
More articles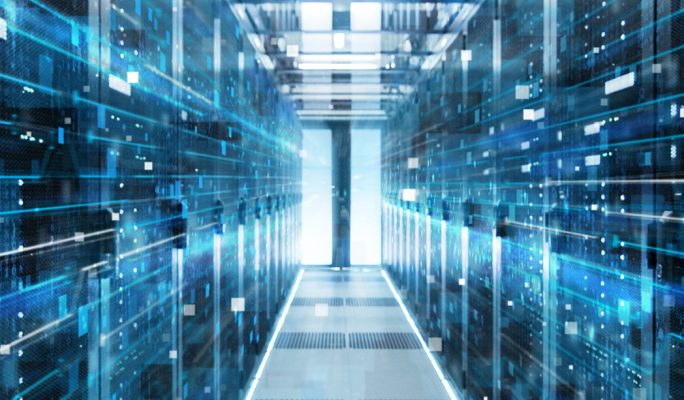
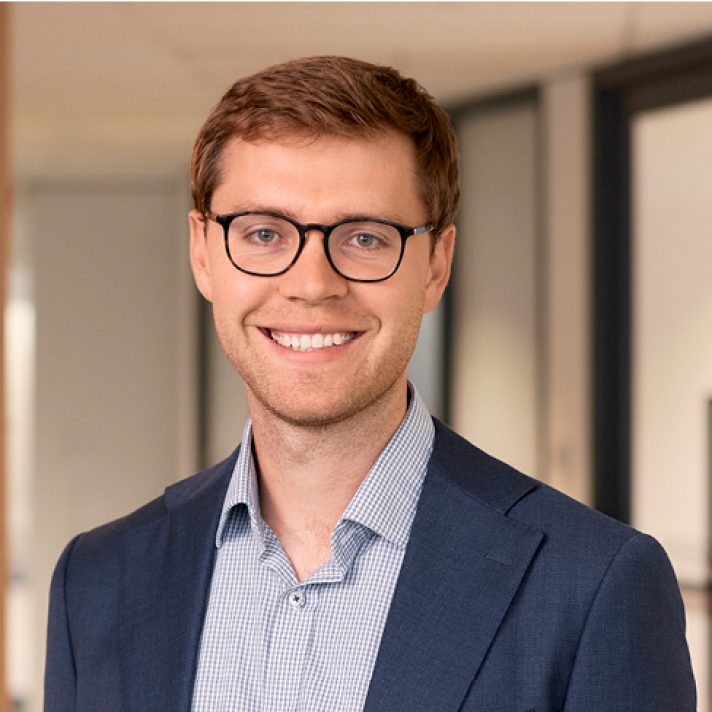
Accuracy and causality the hot topics in data science at SIGKDD 2022
Tom Moulder shares his top picks from the lively discussions at the recent knowledge discovery and data mining conference in Washington DC
Read Article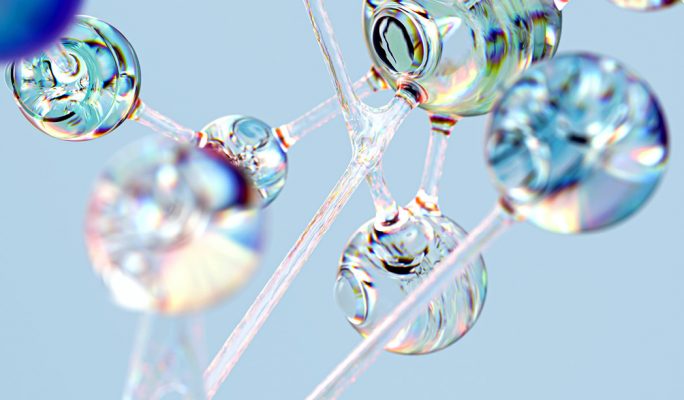
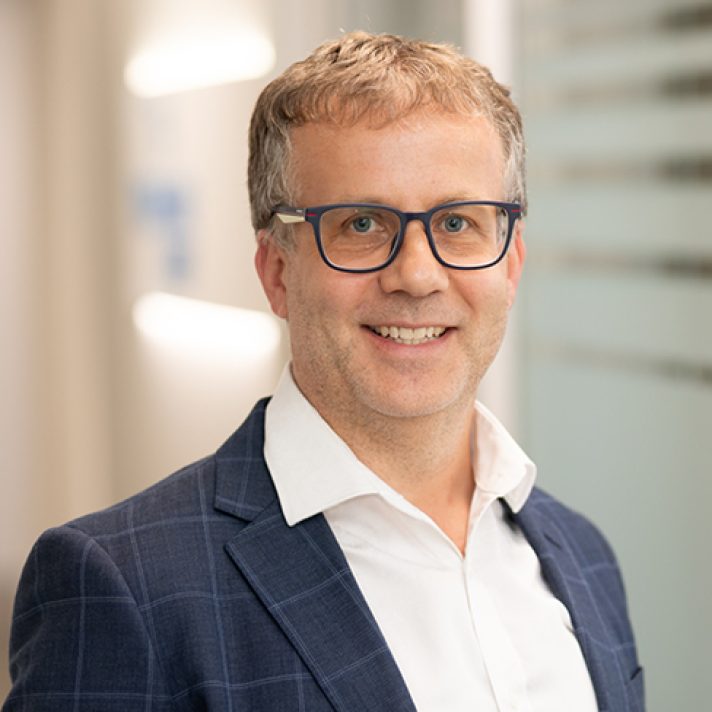
Lessons learned one year on – Algorithm charter for Aotearoa New Zealand
Take a look at the lessons learned from Taylor Fry's newly released review of the Algorithm charter for Aotearoa New Zealand
Read Article